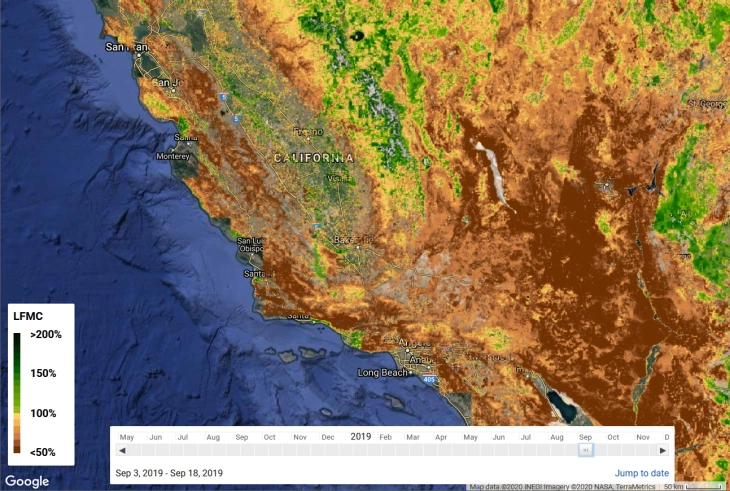
The United States has been plagued by wildfires over the past few years due to climate warming and changing climate patterns. Of course, this natural phenomenon is a serious disaster that cannot be predicted by nature. Researchers at Stanford University have found a way to investigate and predict dry, hazardous areas using machine learning and satellite imaging.
For now, forest fire prediction takes the form of manually collecting branches and leaves to investigate moisture using a forest or shrub area inspection method. It is an accurate and reliable method, but it takes time and is difficult to do on a large scale.
However, if you collect large amounts of ground images and analyze them in detail through ESA’s satellite sentinels and landsets, you can take a data source to assess wildfire risk. Observation methods using satellite imagery existed before, but observation methods differed greatly depending on the place judged by the human eye. Of course it was difficult to investigate a large area. The new method used by the research team allows the use of in-satellite radar to penetrate forests and view images of the bottom surface.
The research team said that this study was conceived by a new type of satellite using a much longer wavelength than before, and through this, it is possible to observe moisture in the depths of the forest and display the moisture content directly. The research team added the data manually measured by the U.S. forestry authorities to new images regularly collected from 2016 and entered it into a machine learning model. This model learns the correlation between various features in an image and ground measurements.
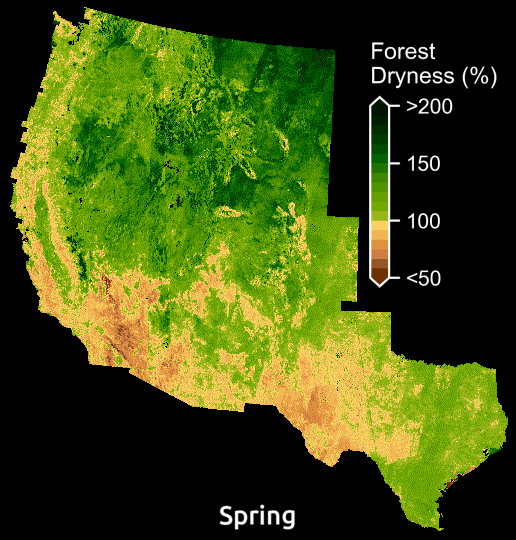
Then, the AI agent obtained this way was tested by forecasting old data that already knew the answer. The results were correct, but most of them were bushlands, one of the most prone to wildfires and one of the largest ecosystems in the western United States. You can also see the dryness forecasts obtained from a yearly model across the West. It’s not a specific help for firefighters, but adding up-to-date data to the same model can provide forecasts for future wildfire seasons and allow authorities to make informed decisions for hazardous areas or safety alerts. Related information here can be found in the (paper here ).
Add comment