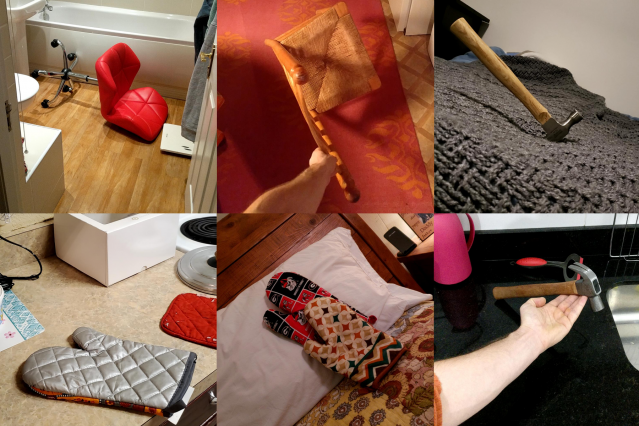
The image recognition model using artificial intelligence aims to accurately identify objects reflected in photos or images. It is intended to be applied to various fields such as autonomous vehicles. For example, in an autonomous vehicle, the object recognition accuracy of the image recognition model is directly related to the safety of the autonomous vehicle, so the dataset used for model learning plays an important role. A team of researchers at MIT and IBM created ObjectNet, a dataset for image recognition models containing various objects.
ObjectNet is a dataset for image recognition models. It does not include the training set used to train the image recognition model, but consists of a test set to verify model accuracy. The number of image test sets included is 50,000, the same as ImageNet.
ImageNet was a data set including images collected through photo sharing services such as Flickr. However, ObjectNet is a dataset that organizes photographic data taken by commissioning freelance photographers for a fee. It is a collection of images that seem to be difficult to recognize images by deliberately tilting the object to the side, shooting from a strange angle that is not usually taken, or by deliberately shooting in a dirty room.
ObjectNet contains pictures that humans may find difficult to judge, such as a chair placed in a dirty room and a picture of the back of the chair. The image recognition model uses a dataset to improve image recognition accuracy through deep learning. However, even in a massive data set such as ImageNet, there is a blind spot in that there are no images such as the back of a chair or a fallen chair in the images contained therein. Therefore, an image recognition model learned from an existing dataset such as ImageNet cannot accurately recognize an image when irregular cases such as the back of a chair or a fallen chair occur.
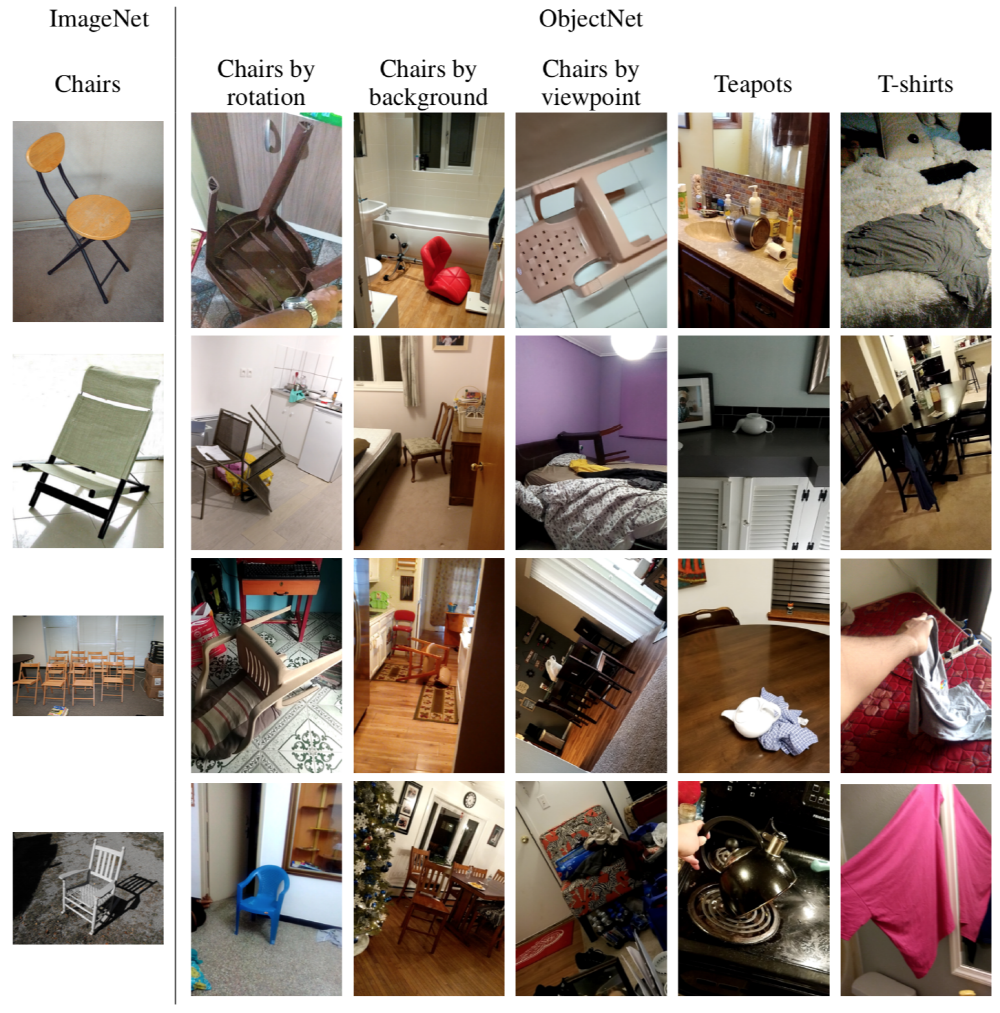
Also, ObjectNet, unlike other datasets, does not contain a training set. Most of the datasets have a separate set of tests for learning the model and verifying accuracy. However, since these two have high similarity, it is said that there are cases where accurate and precise verification cannot be performed.
In fact, as a result of conducting image recognition tests on ImageNet and ObjectNet, ImageNet succeeded in recognizing images with an accuracy of up to 97%, but ObjectNet decreased to 50-55%. This means that the image recognition model, such as the back of an object, is not accurately recognized, and the image recognition model architecture does not include the concept of recognizing the back of an object or a clever angle. In this respect, the image recognition model needs a smarter algorithm.
Experts say that if you want to know how well the algorithm works in the real world, you should test your image recognition model with images that don’t seem biased and you’ve never seen it before. Related information can be found here .
Add comment