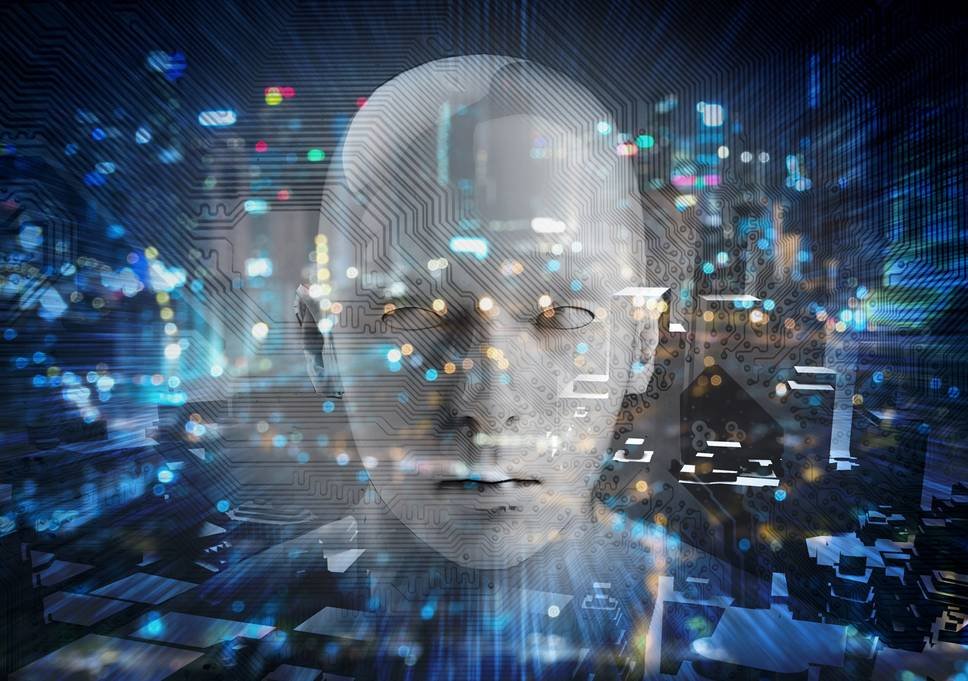
Moore’s Law is the principle that the number of transistors in a semiconductor circuit doubles every 1.5 years. But an expert from NVIDIA’s engineer catches the eye when he says that artificial intelligence is growing rapidly at 5 to 100 times faster than Moore’s Law.
James Wang, an NVIDIA engineer who participated in GeForce Experience and others, says that from 2010 to 2020, the computational processing power required for artificial intelligence learning models is increasing rapidly. From the advent of Perceptron in 1958, until 2010, the processing power doubled every two years. However, from 2010 to 2020, processing power grows at a rate of more than 5 times that of Moore’s Law, 10 times a year. Large companies such as Google’s Alpha Go Zero and Tesla Autopilot expect long-term investment returns and invest enormous budgets on artificial intelligence development, and NVIDIA also deep learning and jointly conduct research and development with universities.
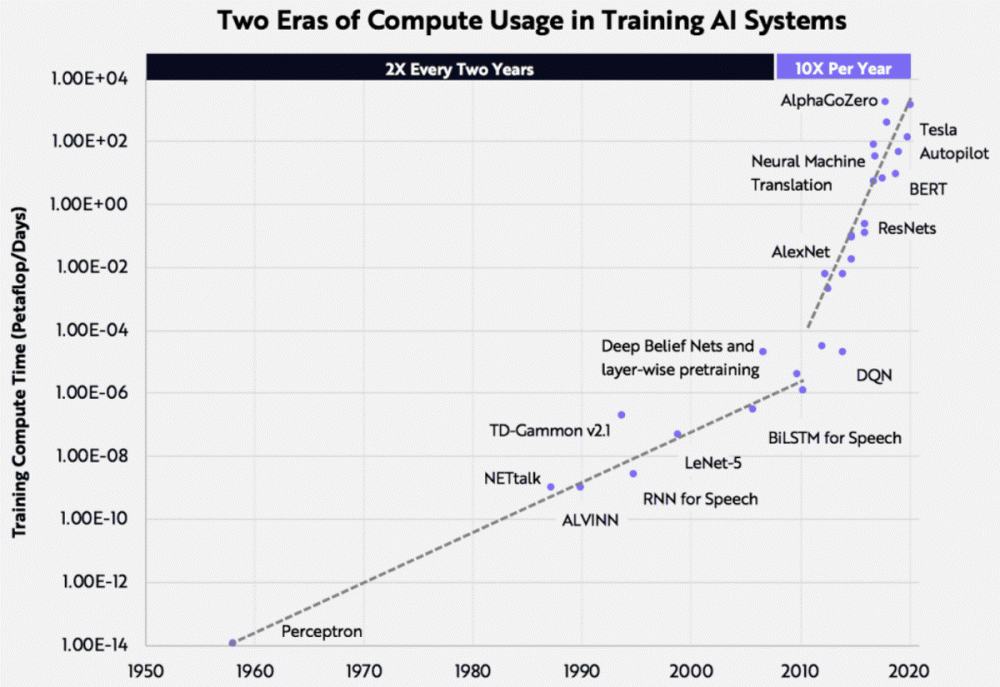
It is also pointed out that the cost of artificial intelligence deep learning is decreasing by a tenth of each year. Around 2017, for example, training an image recognition network like ResNet-50 on the public cloud required around $1,000. But in 2019, the cost has dropped to $10. It is estimated that if the trend of cost reduction is maintained from 2017 to 2019, the cost can go down to $1 in 2020.
Not only that, the cost to sort out a billion photos was $10,000 in 2017, but dropped to just $0.03 in 2020. It is argued that the rate of change in cost related to deep learning is changing 10 to 100 times that of Moore’s Law when compared to Moore’s Law. The reason why the rapid cost reduction was possible is that both hardware and software have evolved dramatically, and between 2017 and 2020, the IC chip and system design technology greatly improved, and hardware dedicated to deep learning appeared.
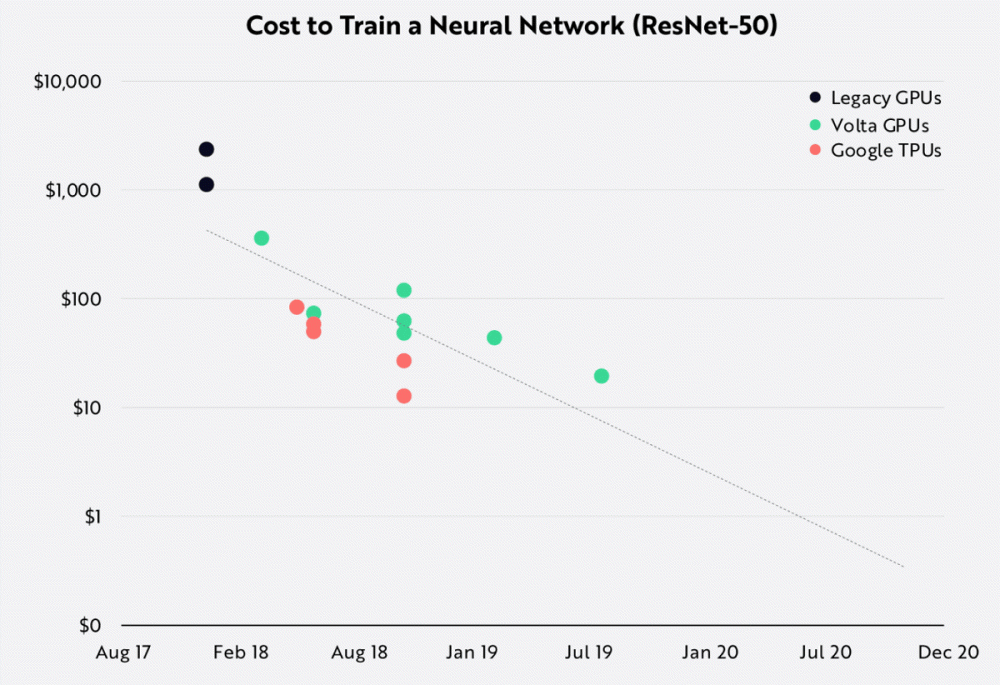
When looking at the performance evaluation of NVIDIA Tesla hardware and software calculated using Stanford University benchmarking, compared to the Tesla K80, the Tesla V100 increased its performance by 16 times. In addition, it is reported that when the Tesla V100 combines the deep learning framework TensorFlow and PyTorch, the software performance is 7 times improved.
Of course, the cost of hardware doesn’t decrease, and it’s also high over the years. For example, NVIDIA’s GPU price for data centers has tripled compared to the previous third generation. Since AWS introduced NVIDIA’s Tesla V100 in 2017, the price of the service has remained constant.
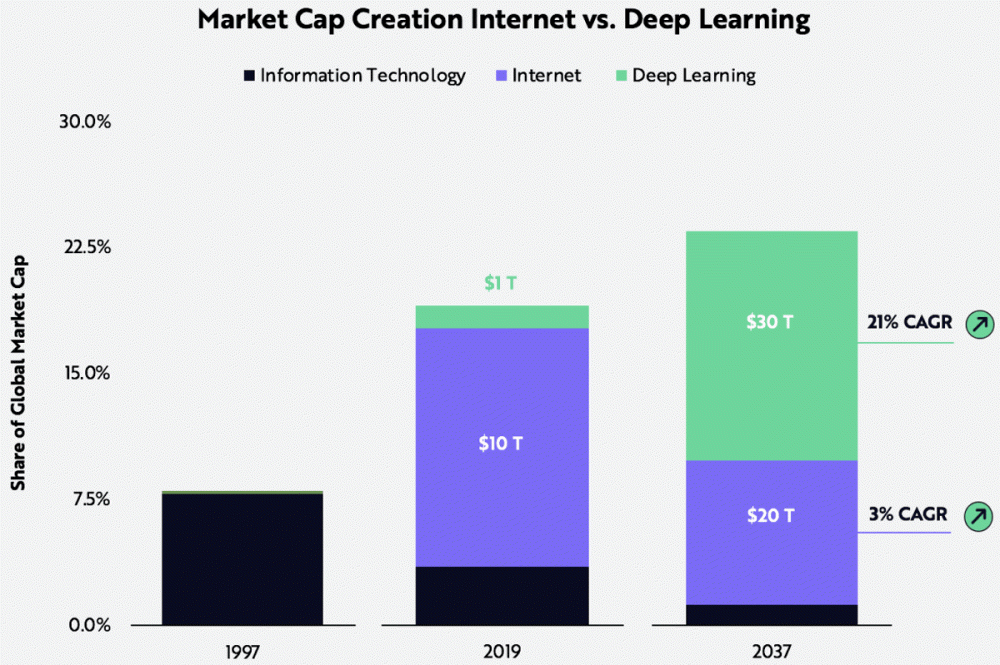
It is also argued that if artificial intelligence technology improves as it is, the market cap of AI-related companies in the global stock market will also increase significantly. As of 2019, artificial intelligence has a market capitalization of about $1 trillion in the global stock market, but it is expected to expand to $30 trillion by 2037 and become a higher market capitalization than Internet-related companies.
It is predicted that AI is still in the process of developing and will likely continue to grow for decades, driven by improved AI computational processing power, the pace of decreasing deep learning costs, and the market capitalization of the stock market. Related information can be found here .
Add comment