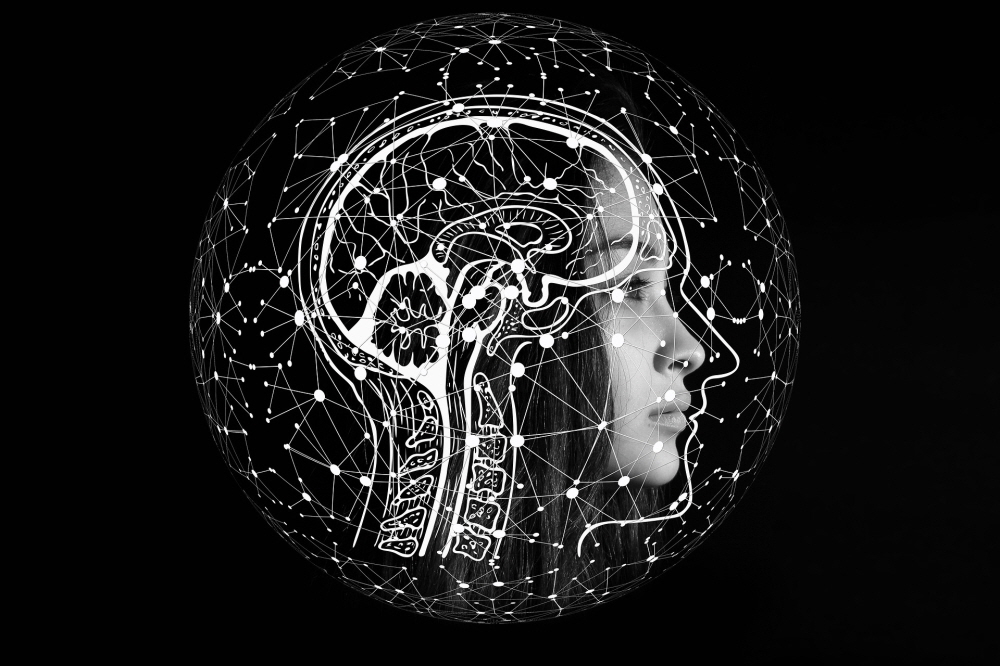
In recent years, AI is driven by a learning method called deep learning in which computers automatically discover data features in large amounts of data without humans touching it. Such deep learning is usually processed by GPU, which is a processor specialized in real-time image processing represented by computer games. However, a researcher at Rice University jointly developed software for CPU that can do deep learning up to 15 times faster than GPUs.
Deep learning is a learning method in which a machine automatically performs the discovery of patterns or rules in data, setting of features, and learning. It has become a technology that leads the field, such as image recognition, translation, and autonomous driving, because it can overcome the limits of human recognition and judgment than the existing methods that humans had to do to discover patterns or rules.
In principle, deep learning performs a matrix integration operation. Meanwhile, a GPU developed for graphics processing is a computing device that performs matrix integration operations that move or rotate three-dimensional graphic polygons. In other words, since GPUs are specialized for redundant operations, they are recently used as optimal processors for deep learning.
However, there is a problem that the GPU is expensive. To solve this problem, a researcher at Rice University paid attention to the deep learning algorithm itself, which currently requires a matrix integration operation. The research team saw deep learning learning as a search problem that can be solved in a hash table, and developed SLIDE (Sub-Linear Deep Learning Engine), an algorithm optimized for the CPU itself. This SLIDE explains that it performs better than GPU-based learning.
The research team emphasized that machine learning using a CPU has an advantage in terms of cost, as CPU is the most popular hardware in computing. The use of SLIDE, which is faithful to matrix operation, achieves the speed of instruction 4-15 times faster than that of the GPU with the CPU. Related information can be found here.
Add comment