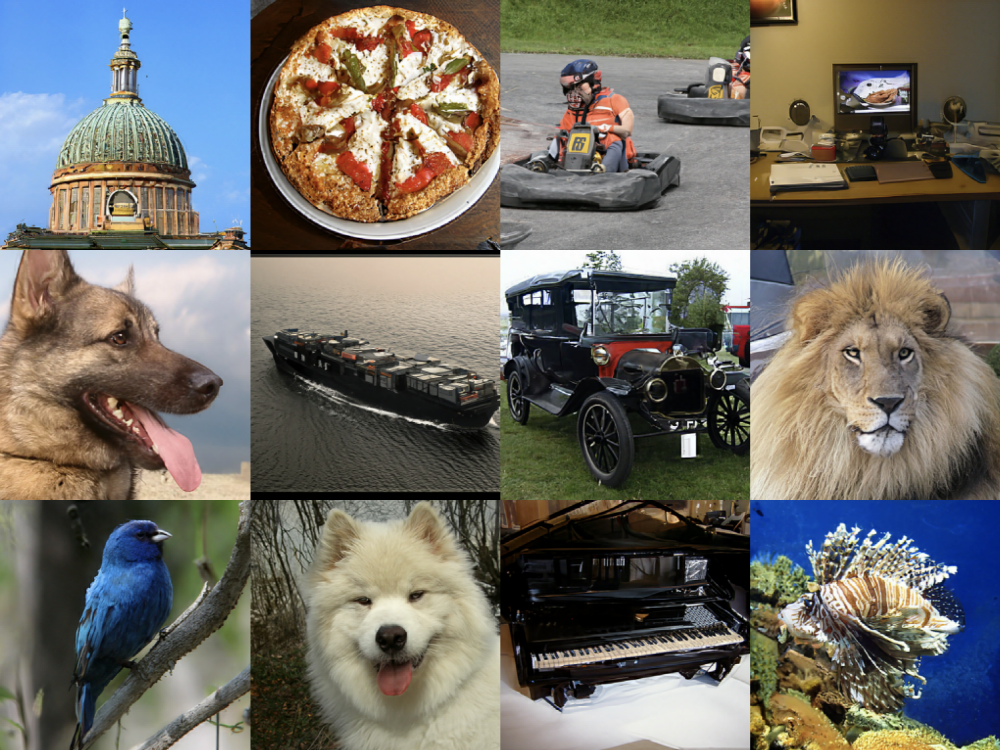
Google AI, a research team at Google, has announced a new approach to improve the diffusion model technique, which adds noise to low-resolution images, processes them until they are pure noise, and creates high-resolution images from there. The technology that generates high-resolution images from low-resolution images with poor image quality is one of the tasks that machine learning is expected to have a wide range of uses, from restoring old photos to improving medical images.
Production models such as adversarial generative networks GANs, VAEs, and autoregression models are usually used to restore high-resolution images from low-resolution images. However, GANs have problems such as collapse that most of the generated images are duplicated, and autoregressive models have problems such as slow synthesis speed.
On the other hand, it is said that the generative model called the diffusion model announced by Google AI in 2015 is being reviewed recently because of its high learning stability and high quality of generated images and voices. Google AI newly announced that it has succeeded in improving the quality of diffusion model image synthesis using two new diffusion model approaches: SR3 (Super-Resolution via Repeated Refinements) and CDM (Cascaded Diffusion Models).
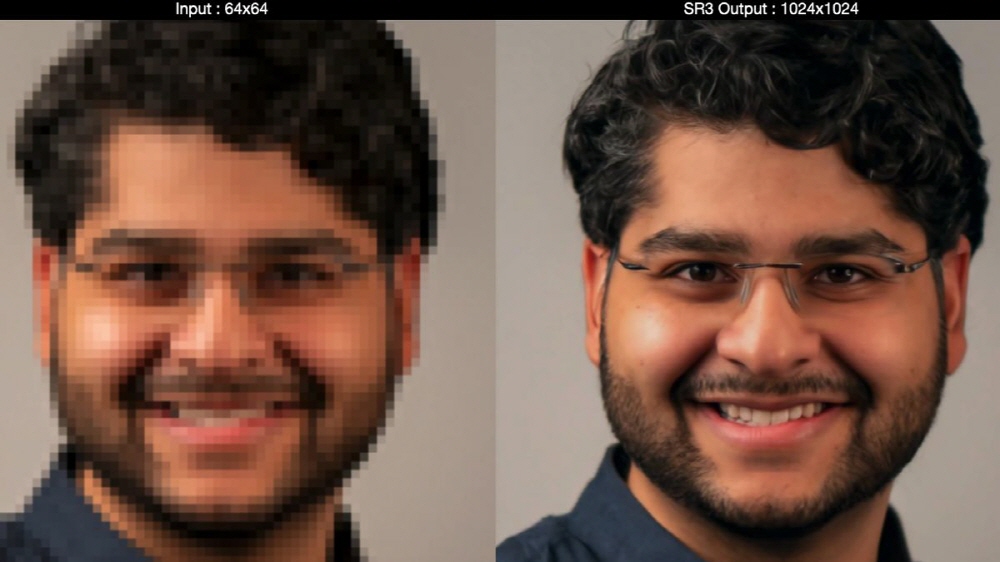
The SR3 first slowly added Gaussian noise to the low-resolution image, damaging it until it was a pure noise image. After that, the neural network reverses the image damage process, removes noise, and creates a high-resolution image that exceeds the original resolution.
In fact, the research team showed images generated by various methods from both the original image and the low-resolution image, and had them determine which is the original image. Compared to FSRGAN (Face Super-Resolution Generative Adversarial Network), PULSE, and regression methods, SR3 has a confusion rate of 47.4% when a 16×16 pixel image is 128×128 pixels.
Google AI is also announcing CDM, a class conditional diffusion model trained on ImageNet, a large image recognition dataset. Because ImageNet contains a variety of datasets, the generative image is likely to be out of sync with the original image, but CDM can produce high-quality images by slowly upscaling the generative model along with label information to multiple spatial resolutions. Related information can be found here.
Add comment