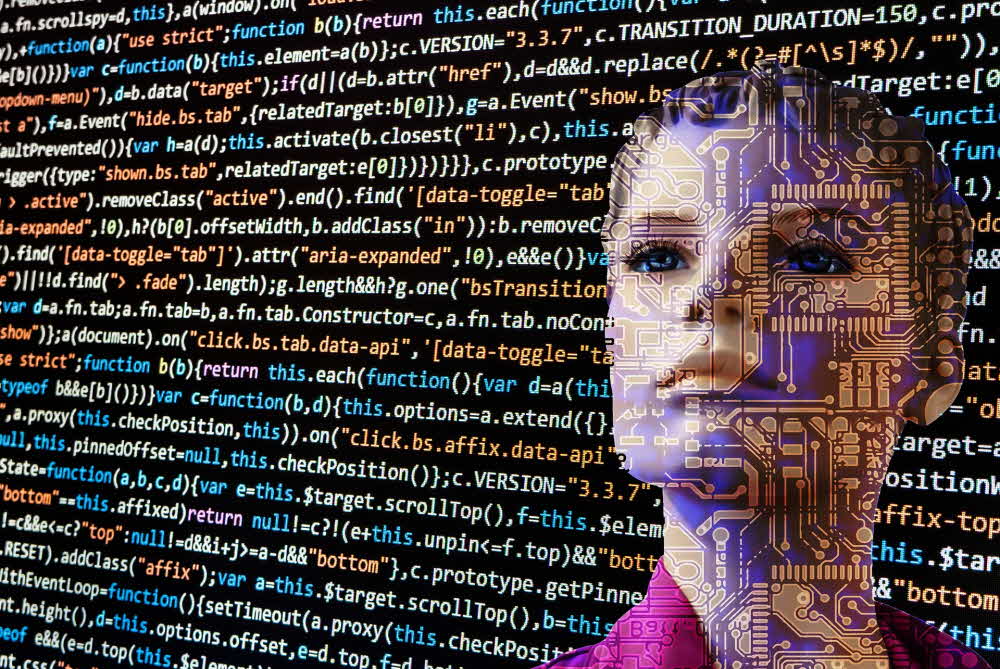
From Google search, shopping, data analysis, and fraud detection, artificial intelligence is helping in various scenes of life. AI is increasing the accuracy of data analysis by repeating machine learning using data set by humans, but if the data used for machine learning is insufficient or if there is prejudice in the data, there is a problem that the prejudice is deepened by learning several times . Bill Simpson-Young, chief executive of the gradient Institute, an AI research institute, is presenting ways to identify the cause of this algorithmic bias and improve the problem.
What they cited as one of the causes of algorithmic bias is poor system design. For example, the AI used by banks to determine where to borrow is typically trained using a dataset of pre-bank loan decisions. The AI is a new loan applicant financier that checks career and employment history and tries to predict whether the applicant will be able to pay off the loan against historical data. However, if past data includes a pattern that bankers rejected loans based on their own bias, AI may learn to make wrong decisions without recognizing this as biased. The prejudice referred to here refers to age, gender, and race, and there is a concern that a prejudice that is rarely seen now will affect AI.
This algorithmic bias poses a great risk to banks. In addition, there is a risk that the decision made by the same AI is repeated, and the pattern of prejudice is exposed to the government and consumers, leading to lawsuits. According to Bill Simpson-Young et al., there are five ways to correct algorithmic bias.
The first is getting better data. Acquire information that has not been brought so far. Bring new data on minority groups and groups that may produce inaccurate results. Second, modify the data set. Do not remove or display information that is considered discriminatory, such as age, gender and race.
The third is to make the model more complex. A simple AI model can make analysis and decisions easier, but it is less accurate and may be based on the majority rather than the few. Fourth, system change. The AI system can change the control pattern in advance. It can be adjusted to ignore algorithmic bias by setting different thresholds for the prime group. Finally, the prediction model was changed. Setting up an appropriate predictive model helps reduce algorithmic bias.
Prof. Bill Simpson-Young argues that governments and corporations seeking to adopt AI-driven decision-making should consider the general principles of fairness and human rights, and argue that systems need to be carefully designed and monitored to avoid false results caused by algorithmic bias. It is revealed that there is a need to build a more fair society as well as improve productivity as decision-making by AI is becoming common. Related information can be found here .
Add comment